Departamento de Relaciones Económicas Internacionales
Artículos
Remarks on the interaction between beliefs, perceptions, and economics forecasting
Esteban Javier Leguizamón[1]
Juan Manuel Ottaviani[2]
This document is the result of the authors’ debate regarding the macroeconomic performance of different economies based on different forecasts used as inputs. Our “contribution” is not an original and untreated topic. Instead, we follow a line of argument while organizing the conclusions and ideas of previous authors. A positive externality found in this document is that expressing abstract ideas in this way makes them understandable for professionals who do not have a deep knowledge of economics. The following pages discuss forecasting failures in economics, the main reasons why an exogenous shock’s intrinsic nature is almost uncertain, and how the beliefs and perceptions of economic agents are permeable to new information. Thus, their expectations and decision-making processes may be influenced, and coordinated behavioral patterns may emerge from new «trustworthy» data.
Economic agents, especially policymakers, require dependable information on the present state of the economy and its anticipated future performance. Unfortunately, they usually possess only inadequate data for decision-making. The leading global economic institutions, including the IMF, the World Bank, and the International and Development Bank, closely track the global economy’s performance and release a range of figures to address this void. Precise forecasting becomes crucial when authorities face an economic crisis. To take informed decisions, policymakers, and economic agents require a steady stream of concise and reliable information. Economic forecasting is carried out by these institutions and professionals in the private sector. Forecasting exercises serve not only as a source of information for governments and key economic agents regarding the economy’s performance but also to coordinate expectations and beliefs. Providing this information can subtly affect economic agents’ decisions, encourage economic growth, and create a self-fulfilling prophecy.
Most institutions use Dynamic Stochastic General Equilibrium (DSGE) models for their forecasts. This means that in their projections, economic agents make decisions based on the Full Information Rational Expectations (FIRE) framework. Heymann and Pascuini (2021 p.2), explain:
“[…]in Macroeconomics, the notion of Rational expectations is an ambiguous, ill-defined concept, which, when given a specific definition, varies between (i) the statement that the probability distributions of the outcomes anticipated by agents equal the objective distributions characterizing in fact those outcomes and (ii) the idea that the expectations of agents incorporate at each moment the knowledge derived from economic analysis”.
The authors also remark that it is feasible to specify the state of the system as a function of two main sequences:
- The realized history (past realizations of relevant variables).
- The looking ahead behavior of economic agents.
It is worth noting that agents perceive the environment (i.e., information regarding the past, and present realizations of the variables, news, other agents’ decisions, etc.) process the information they gather, and form their expectations and beliefs. After the process, they make economic decisions that may affect the system’s behavior and their perceptions. This implies that forecasts performed by international institutions affect both how agents perceive relevant information about the system status and how they modify their decision process. Weitzmann (2007) argues that in a nonergodic situation, everyone is perennially uncertain about the current structure of the economic system. His argument implies that the usage of past realizations (values) of relevant variables in forecasting exercises may lead to systematic biases (defined as the difference between the forecasted value of a variable and its realization). Therefore, agents are constantly learning about the world they live in, and the system will not converge to a FIRE equilibrium. The reason is that no matter how the information they perceive is filtered or processed, there is no fixed point to converge. West (2017) concisely describes this phenomenon: “It is a complex adaptive system of complex adaptive systems”.
Taking into consideration the former definition, the following question arises: what is truly exogenous in an environment where agents know the objective distribution function of perturbances? The question implies that for a truly exogenous shock to happen, not only economic agents must be unable to anticipate it, but it also cannot belong to any possible state of the economy as they know it (having each state an associated probability different than zero). If so, this means that in this kind of ever-evolving non-stationary market economy, it is impossible to have full knowledge of the space of states.
Guzmán and Stiglitz (2020) introduce a framework to analyze this kind of situation. They propose analyzing economic downturns through the lens of a dynamic disequilibrium theory with randomness. The setup introduced by the authors is based on thinking of the economy as a system in constant evolution with at least two fundamental characteristics:
- Uncertainty about the future of the system, understood as a random evolutionary path. Note that even if the “representative” agent knows the probability of occurrence of the possible states of the system he knows, exists a subset of possible states of the world (system) which he is always unable to know about.
Assuming:
Let be the number of possible states of the world known by agent during time (i.e., is the number of elements that belong to the set ), to ensure the agent’s continual learning and the finiteness of the number of possible states of nature n, the following condition serves as a boundary for the speed of the agent’s learning rate:
This condition ensures that the agent is unable to know all the possible states of the world and his learning rate is bounded by the rate of expansion of . It follows that it becomes impossible for him to achieve perfect accuracy in his forecasts.
According to Guzmán and Stiglitz (2020 p.20):
“The fact that macroeconomic inconsistencies are partly a subjective matter centers attention on the beliefs of market participants. A change in beliefs is a perturbation that is not really a “shock” in the standard sense, but the consequence of a social process of formation of beliefs about the economy in an evolving environment with limited knowledge and information, in which there are feedback effects in the learning process between the reality and what the agents think they know about the economy. In fact, the word “shock” is not helpful here. The most meaningful changes in beliefs—the ones we focus on—are endogenous. So too are the major perturbations that have afflicted capitalism—the breaking of a credit bubble, a tulip bubble, a housing bubble, the tech bubble, or the massive change in beliefs provoked by the COVID-19 shock that led to radical changes in the organization of the economic system that had not been even envisioned as a possibility.”
In this way, bounded rationality has to be introduced to allow for purely exogenous “shocks” to happen.
Forecasting experiences in the global economy since the COVID-19 shock:
The process of economic recovery, especially after large shocks that may generate changes in capital accumulation and relations of production, is a highly particular phenomenon that challenges economists’ forecasting practices. Usually, forecasting is based on past realizations of the most relevant variables of the economic system, how they relate and influence each other, and some assumption is introduced regarding how economic agents perceive information about the environment and the decision-making process they follow.
As the dawn of the aftermath of the COVID-19 pandemic emerged, the global economy commenced its recovery from the recession triggered by the lockdowns and quarantines. Precisely when it was gaining momentum and reorganizing itself to address urgent relegated problems such as poverty, immigration, education, energy, and food production, etc. At this critical juncture, the conflict between Russia and Ukraine struck. Another straight punch that undoubtedly presents a formidable challenge to international institutions, the global economy, and professional econometricians dedicated to forecasting exercises as well.
Figure 1 shows five different series of the World’s GDP per capita series produced by the IMF (2018 GDP per capita value equals 100). The green line was the last forecast made by the institution before the COVID-19 shock, it is built using the 2019 growth rate of the series and the forecasted values for all the available periods after 2019. On the other side, the dark blue line is the one that shows the real behavior of the per capita GDP series until April 2023 (the last WEO published) and includes forecasted values only for the period 2023-2027.
Figure 1 – GDP per capita forecasted values by the IMF in different WEO publications
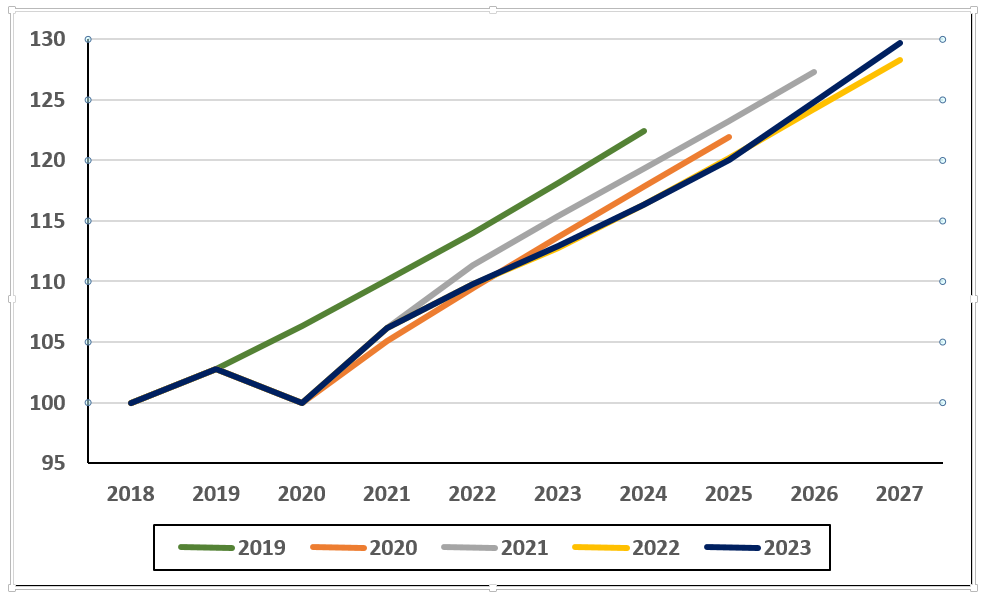
Source: Author’s elaboration based on World Economic Outlook (IMF).
The following facts must be highlighted:
- The green line that represents the forecasted values before the COVID-19 shock, leaves no space for any doubts about the unexpected character of it.
- The orange line was built with real per capita GDP variations until 2020 and the forecasted values thereafter. It shows that until 2021 there were not any expectations or signs of a robust economic recovery. The slope of the green and orange lines is almost the same, despite being the orange one forecasted at least one year after the green pre-COVID values.
- Finally, it is worth noticing that after massive vaccination started in 2021, the light grey line shows that forecasts were revised up wise almost immediately. When looking at the yellow and dark blue lines, it becomes clear that the grey line showing the 2021 forecasts, reveals the economic agent’s overreaction to the vaccines. Trends were revised downwards in the following WEO publication.
In 2020, the growth rate was projected to be -4.36%, but the actual economic contraction was -2.81%, which is somewhat surprising. While anyone can easily locate past projections made by the IMF or any other institution and demonstrate their forecasting errors, what lesson can analysts learn from these past failures?
Estimators usually are means, medians, or other moments of a distribution. When the majority of punctual forecasts end up being biased or erroneous, an improvement should be the usage of confidence intervals. An uncertain environment enhances the value of confidence intervals which can be state dependent. Therefore, confidence intervals could provide a more accurate picture than trying to pin down specific values. By doing so, this approach offers a significant edge for decision-making, enabling policymakers and investors to weigh the potential risks of different courses of action, based on a range of possible outcomes.
In Milton Friedman’s Nobel Memorial lecture, “Inflation and Unemployment”, he highlights the importance of understanding correlations, empirical regularities, and causation: “In order to recommend a course of action to achieve an objective, we must first know whether that course of action will in fact promote the objective” (Friedman, 1976 p.2).
Final remarks
Forecasting in social sciences is undoubtedly a complex task. The evolving nature of the system implies that data related to a “long” period in the past is almost noninformative about the present behavior of the economy (Weitzmann, 2007). Analysts must be careful about conclusions and the procedure followed in the forecasting exercise when communicating the limitations of the results to policymakers. It becomes clear that if the accuracy of any forecasting exercise is heavily dependent on the inputs (data) used and the assumptions made, extreme precaution must be the rule in the usage of the results. Therefore, in a context where key variables are constantly evolving and “shocks” are more common than economists thought years ago, decisions that rely on those forecasts must be analyzed multiple times from multiple perspectives before following any course of action. As Heymann and Pascuini (2021 p.12) argue the “state of the art” understood as the model proposed by professional economists, can not be (by construction) the ultimate instrument to generate expectations.
Finally, a balance between economic theory, forecasting, and profound knowledge of the actual state of the economic system is the best “weapon” experts have to perform their an analysis and “predictions” while recognizing their own limitations.
Bibliographic references
Guzman, M., & Stiglitz, J. E. (2020). Towards a dynamic disequilibrium theory with randomness. Oxford Review of Economic Policy, 36(3), 621-674.
Heymann, D., & Pascuini, P. (2021). On the (in) consistency of RE modeling.
Timmermann, A. (2007). An evaluation of the World Economic Outlook forecasts. IMF Staff Papers, 54(1), 1-33.
Long, A., & Ascent, D. (2020). World economic outlook. International Monetary Fund, 177.
Schuschny, A. (2001) Auto organización en sistemas económicos. Available at http://bibliotecadigital.econ.uba.ar/econ/collection/tesis/document/1501-1161_SchuschnyAR
Weitzman, M. L. (2007). Subjective expectations and asset-return puzzles. American Economic Review, 97(4), 1102-1130.
[1] Licenciado en Economía por la Universidad Nacional de Rosario, Magíster en Economía por la Universidad Nacional de La Plata, y candidato a Doctor en Economía (UNLP). Es docente de Relaciones Económicas Internacionales II en el IRI y de Macroeconomía I, en la FCE de la UNLP. Coordinador del Departamento de Relaciones Económicas Internacionales (IRI-UNLP).
[2] Estudiante avanzado de la Licenciatura en Economía de la Facultad de Ciencias Económicas de la UNLP, Ayudante de cátedra de la asignatura Macroeconomía I, FCE.UNLP.